Understanding Stateful LSTM Recurrent Neural Networks in Python with Keras
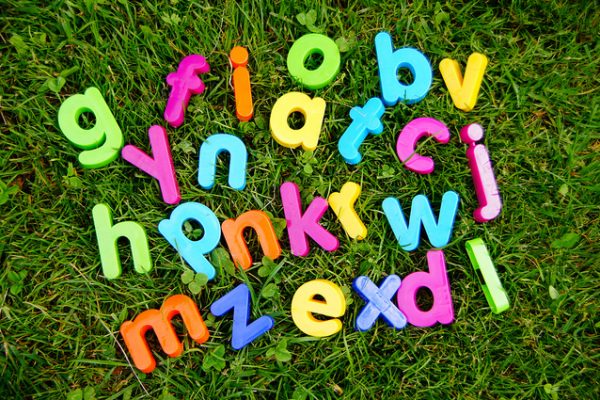
Last Updated on August 27, 2020
A powerful and popular recurrent neural network is the long short-term model network or LSTM.
It is widely used because the architecture overcomes the vanishing and exposing gradient problem that plagues all recurrent neural networks, allowing very large and very deep networks to be created.
Like other recurrent neural networks, LSTM networks maintain state, and the specifics of how this is implemented in Keras framework can be confusing.
In this post you will discover exactly how state is maintained in LSTM networks by the Keras deep learning library.
After reading this post you will know:
- How to develop a naive LSTM network for a sequence prediction problem.
- How to carefully manage state through batches and features with an LSTM network.
- How to manually manage state in an LSTM network for stateful prediction.
Kick-start your project with my new book Deep Learning With Python, including step-by-step tutorials and the Python source code files for all examples.
Let’s get started.
- Update Mar/2017: Updated example for Keras 2.0.2, TensorFlow 1.0.1 and Theano 0.9.0.
- Update Aug/2018: Updated exampels for Python 3, updated stateful example to get 100% accuracy.
- Update Mar/2019: Fixed typo in the stateful
To finish reading, please visit source site