Understand Your Problem and Get Better Results Using Exploratory Data Analysis
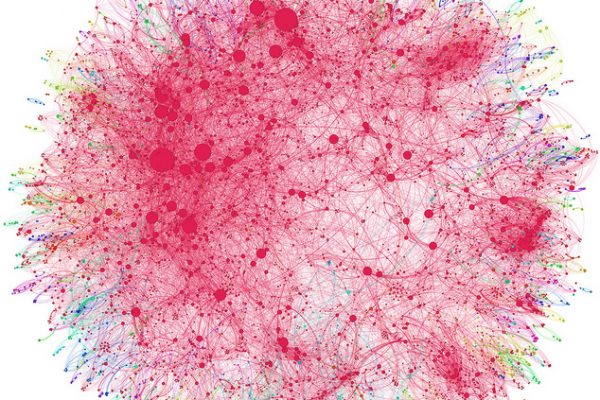
Last Updated on August 15, 2020
You often jump from problem-to-problem in applied machine learning and you need to get up to speed on a new dataset, fast.
A classical and under-utilised approach that you can use to quickly build a relationship with a new data problem is Exploratory Data Analysis.
In this post you will discover Exploratory Data Analysis (EDA), the techniques and tactics that you can use and why you should be performing EDA on your next problem.

Exploratory Data Analysis
Photo by Andy Lamb, some rights reserved
Build a Relationship with the Data
The process of classical statistics is to test hypotheses already held about the problem.
This is done by fitting specific models and demonstrating specific relationships in the data. It’s an effective approach, but it assumes you already have hypotheses about the problem, that you already understand the data. This is rarely the case in applied machine learning.
Before you can model the data and test your hypotheses, you need to build a relationship with the
To finish reading, please visit source site