Tune Learning Rate for Gradient Boosting with XGBoost in Python
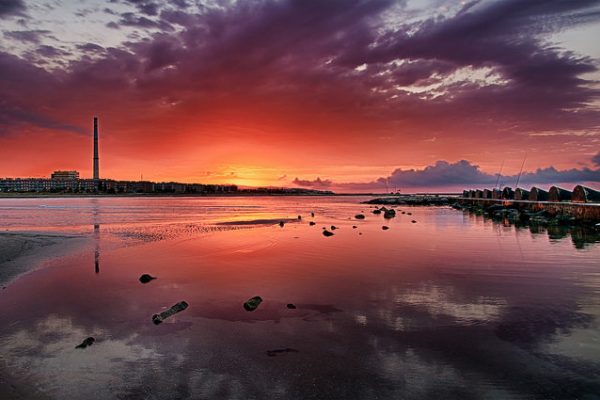
Last Updated on August 27, 2020
A problem with gradient boosted decision trees is that they are quick to learn and overfit training data.
One effective way to slow down learning in the gradient boosting model is to use a learning rate, also called shrinkage (or eta in XGBoost documentation).
In this post you will discover the effect of the learning rate in gradient boosting and how to tune it on your machine learning problem using the XGBoost library in Python.
After reading this post you will know:
- The effect learning rate has on the gradient boosting model.
- How to tune learning rate on your machine learning on your problem.
- How to tune the trade-off between the number of boosted trees and learning rate on your problem.
Kick-start your project with my new book XGBoost With Python, including step-by-step tutorials and the Python source code files for all examples.
Let’s get started.
- Update Jan/2017: Updated to reflect changes in scikit-learn API version 0.18.1.

Tune Learning Rate
To finish reading, please visit source site