Neural Representations for Modeling Variation in English Speech
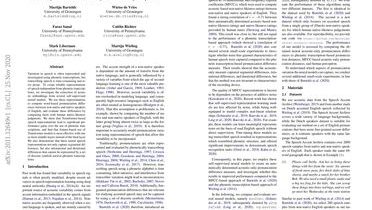
Variation in speech is often represented and investigated using phonetic transcriptions, but transcribing speech is time-consuming and error prone. To create reliable representations of speech independent from phonetic transcriptions, we investigate the extraction of acoustic embeddings from several self-supervised neural models...
We use these representations to compute word-based pronunciation differences between non-native and native speakers of English, and evaluate these differences by comparing them with human native-likeness judgments. We show that Transformer-based speech representations lead to significant performance gains over the use of phonetic transcriptions, and find that feature-based use of Transformer models is most effective