Inverting Gradients – How easy is it to break privacy in federated learning?
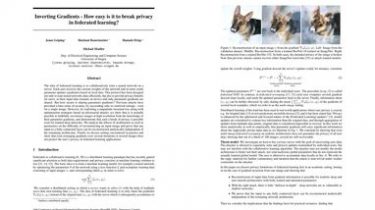
The idea of federated learning is to collaboratively train a neural network on a server. Each user receives the current weights of the network and in turns sends parameter updates (gradients) based on local data...
This protocol has been designed not only to train neural networks data-efficiently, but also to provide privacy benefits for users, as their input data remains on device and only parameter gradients are shared. But how secure is sharing parameter gradients? Previous attacks have provided a false sense of security, by succeeding only in contrived settings – even for a single image.