Implementation of Trajectory Transformer with attention caching and batched beam search
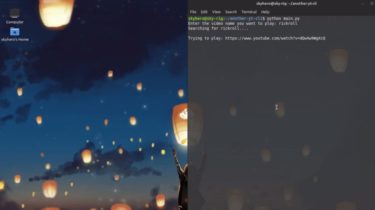
This is reimplementation of Trajectory Transformer, introduced in Offline Reinforcement Learning
as One Big Sequence Modeling Problem paper.
The original implementation has few problems with inference speed, namely quadratic attention during
inference and sequential rollouts. The former slows down planning a lot, while the latter does not
allow to do rollouts in parallel and utilize GPU to the full.
Still, even after all changes, it is not that fast compared to traditional methods such as PPO or SAC/DDPG.
However, the gains are huge, what used to take hours now takes a dozen minutes (25 rollouts, 1k steps each, for example).
Training time remains the same, though.
Changes
Attention caching
During beam search we’re