Image Inpainting with Learnable Feature Imputation
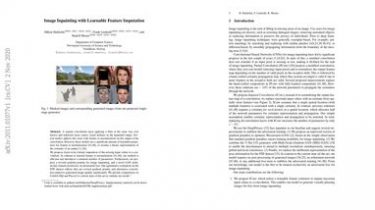
A regular convolution layer applying a filter in the same way over known and unknown areas causes visual artifacts in the inpainted image. Several studies address this issue with feature re-normalization on the output of the convolution...
However, these models use a significant amount of learnable parameters for feature re-normalization, or assume a binary representation of the certainty of an output. We propose (layer-wise) feature imputation of the missing input values to a convolution. In contrast to learned feature re-normalization, our method is efficient and introduces a minimal number of parameters. Furthermore, we propose a revised