How to Diagnose Overfitting and Underfitting of LSTM Models
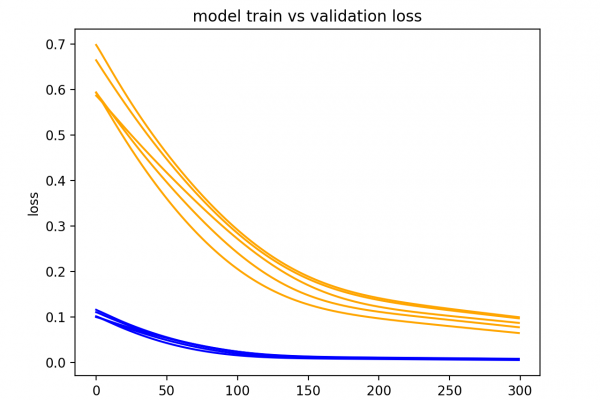
Last Updated on January 8, 2020
It can be difficult to determine whether your Long Short-Term Memory model is performing well on your sequence prediction problem.
You may be getting a good model skill score, but it is important to know whether your model is a good fit for your data or if it is underfit or overfit and could do better with a different configuration.
In this tutorial, you will discover how you can diagnose the fit of your LSTM model on your sequence prediction problem.
After completing this tutorial, you will know:
- How to gather and plot training history of LSTM models.
- How to diagnose an underfit, good fit, and overfit model.
- How to develop more robust diagnostics by averaging multiple model runs.
Kick-start your project with my new book Long Short-Term Memory Networks With Python, including step-by-step tutorials and the Python source code files for all examples.
Let’s get started.
- Update Jan/2020: Updated API for Keras 2.3 and TensorFlow 2.0.
Tutorial Overview
This tutorial is divided into 6 parts; they are:
- Training History in Keras
- Diagnostic Plots
- Underfit Example
- Good Fit Example
- Overfit Example
- Multiple Runs Example