Graph Neural Networks meet Personalized PageRank
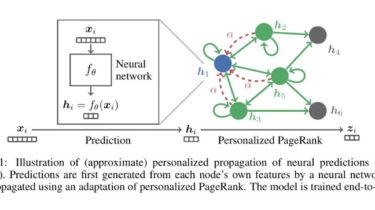
APPNP
A PyTorch implementation of Predict then Propagate: Graph Neural Networks meet Personalized PageRank (ICLR 2019).
Abstract
Neural message passing algorithms for semi-supervised classification on graphs have recently achieved great success. However, these methods only consider nodes that are a few propagation steps away and the size of this utilized neighborhood cannot be easily extended. In this paper, we use the relationship between graph convolutional networks (GCN) and PageRank to derive an improved propagation scheme based on personalized PageRank. We utilize this propagation procedure to construct personalized propagation of neural predictions (PPNP) and its approximation, APPNP. Our model’s training time is on par or faster and its number of parameters on par or lower than previous models. It leverages a large, adjustable neighborhood for classification and