Gradient Boosting with Scikit-Learn, XGBoost, LightGBM, and CatBoost
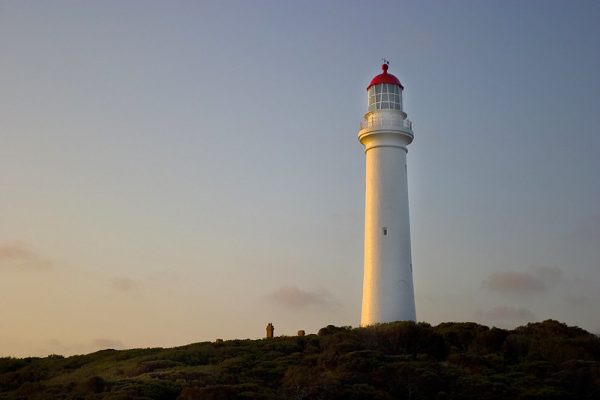
Last Updated on August 28, 2020
Gradient boosting is a powerful ensemble machine learning algorithm.
It’s popular for structured predictive modeling problems, such as classification and regression on tabular data, and is often the main algorithm or one of the main algorithms used in winning solutions to machine learning competitions, like those on Kaggle.
There are many implementations of gradient boosting available, including standard implementations in SciPy and efficient third-party libraries. Each uses a different interface and even different names for the algorithm.
In this tutorial, you will discover how to use gradient boosting models for classification and regression in Python.
Standardized code examples are provided for the four major implementations of gradient boosting in Python, ready for you to copy-paste and use in your own predictive modeling project.
After completing this tutorial, you will know:
- Gradient boosting is an ensemble algorithm that fits boosted decision trees by minimizing an error gradient.
- How to evaluate and use gradient boosting with scikit-learn, including gradient boosting machines and the histogram-based algorithm.
- How to evaluate and use third-party gradient boosting algorithms, including XGBoost, LightGBM, and CatBoost.
Let’s get started.
