Exploring Dynamic Context for Multi-path Trajectory Prediction
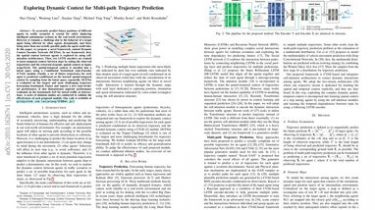
To accurately predict future positions of different agents in traffic scenarios is crucial for safely deploying intelligent autonomous systems in the real-world environment. However, it remains a challenge due to the behavior of a target agent being affected by other agents dynamically, and there being more than one socially possible paths the agent could take...
In this paper, we propose a novel framework, named Dynamic Context Encoder Network (DCENet). In our framework, first, the spatial context between agents is explored by using self-attention architectures. Then, two LSTM encoders are trained to learn temporal context between steps