Essence of Bootstrap Aggregation Ensembles
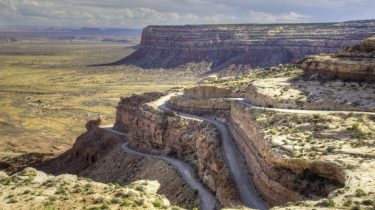
Bootstrap aggregation, or bagging, is a popular ensemble method that fits a decision tree on different bootstrap samples of the training dataset.
It is simple to implement and effective on a wide range of problems, and importantly, modest extensions to the technique result in ensemble methods that are among some of the most powerful techniques, like random forest, that perform well on a wide range of predictive modeling problems.
As such, we can generalize the bagging method to a framework for ensemble learning and compare and contrast a suite of common ensemble methods that belong to the “bagging family” of methods. We can also use this framework to explore further extensions and how the method can be further tailored