A Gentle Introduction to Expected Value, Variance, and Covariance with NumPy
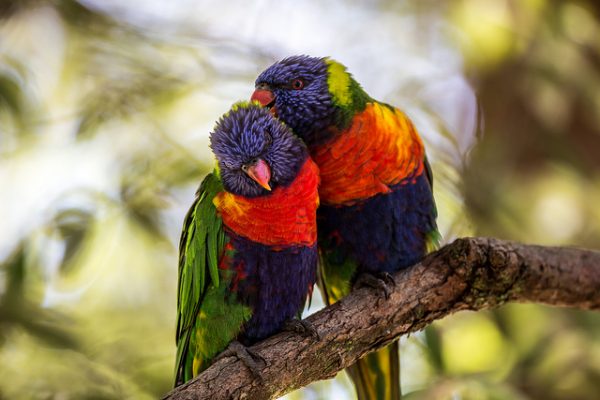
Last Updated on November 16, 2019
Fundamental statistics are useful tools in applied machine learning for a better understanding your data.
They are also the tools that provide the foundation for more advanced linear algebra operations and machine learning methods, such as the covariance matrix and principal component analysis respectively. As such, it is important to have a strong grip on fundamental statistics in the context of linear algebra notation.
In this tutorial, you will discover how fundamental statistical operations work and how to implement them using NumPy with notation and terminology from linear algebra.
After completing this tutorial, you will know:
- What the expected value, average, and mean are and how to calculate them.
- What the variance and standard deviation are and how to calculate them.
- What the covariance, correlation, and covariance matrix are and how to calculate them.
Kick-start your project with my new book Linear Algebra for Machine Learning, including step-by-step tutorials and the Python source code files for all examples.
Let’s get started.
- Updated Mar/2018: Fixed a small typo in the result for vector variance example. Thanks Bob.
