A Domain Adaption Transfer Learning Bearing Fault Diagnosis Model Based on Wide Convolution Deep Neu
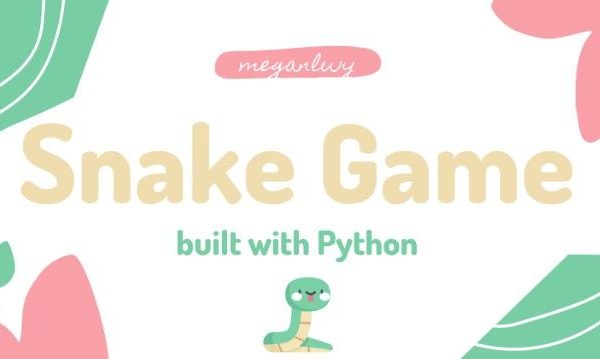
In the context of intelligent manufacture, advanced health diagnostic systems are urgently needed for factory machinery and equipment. Bearing is an important part of contemporary mechanical equipment, and its health condition directly affects the stability and safety of the operation of the equipment it is located in, so the early diagnosis of bearing failure is of great significance. However, traditional intelligent diagnostic methods cannot continue to maintain good fault diagnosis capabilities under changing workloads. Inspired by the idea of transfer learning, a combined approach is proposed. In the method, Deep Convolutional Neural Networks with Wide First-layer Kernel is used to extract features to classify the health conditions. Domain adaptation maximizes the loss of the domain classifier to confuse the source domain dataset with the target domain dataset, and finally