Classification-based Quality Estimation: Small and Efficient Models for Real-world Applications
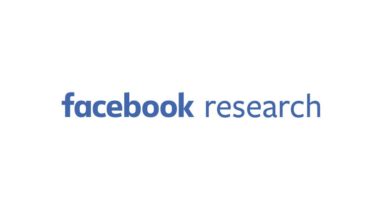
Abstract
Sentence-level Quality Estimation (QE) of machine translation is traditionally formulated as a regression task, and the performance of QE models is typically measured by Pearson correlation with human labels. Recent QE models have achieved previously-unseen levels of correlation with human judgments, but they rely on large multilingual contextualized language models that are computationally expensive and thus infeasible for many real-world applications. In this work, we evaluate several model compression techniques for QE and find that, despite their popularity